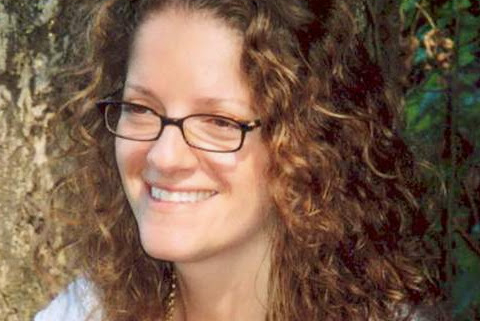
Frontiers in Biostatistics Seminar
Tuesday December 5, 2023 @ 1pm ET
YouTube Link
Malka Gorfine, PhD
Professor, Department of Statistics, Tel Aviv University, Israel
Abstract: Massive sized survival datasets become increasingly prevalent with the development of the healthcare industry, and pose computational challenges unprecedented in traditional survival analysis use cases. In this work we analyze the UK-biobank colorectal cancer data with genetic and environmental risk factors, including a time-dependent coefficient, which transforms the dataset into “pseudo-observation” form, thus critically inflating its size. A popular way for coping with massive datasets is downsampling them, such that the computational resources can be afforded by the researcher. Cox regression has remained one of the most popular statistical models for the analysis of survival data to-date. This work addresses the settings of right censored and possibly left-truncated data with rare events, such that the observed failure times constitute only a small portion of the overall sample. We propose Cox regression subsampling-based estimators that approximate their full-data partial-likelihood-based counterparts, by assigning optimal sampling probabilities to censored observations, and including all observed failures in the analysis. The suggested methodology is applied on the UK-biobank for building a colorectal cancer risk-prediction model, while reducing the computation time and memory requirements. We establish asymptotic properties under suitable conditions and develop a framework for determining the optimal subsample size.
Want to get our weekly events newsletter? Click here!