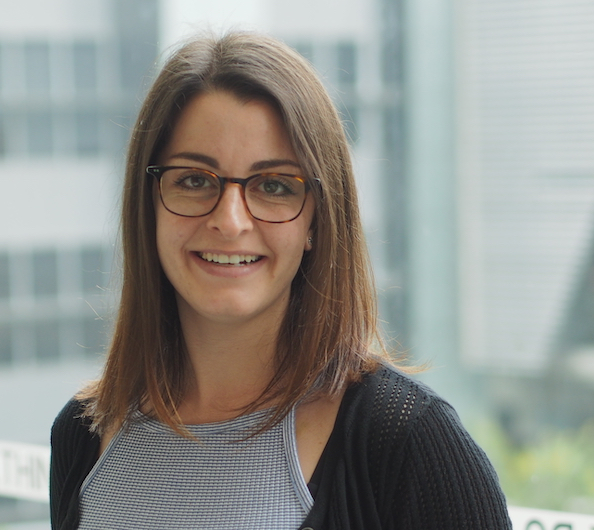
Frontiers in Biostatistics Webinar
Marianne Menictas
Postdoctoral Fellow, Department of Statistics
Harvard University
Mobile health (mHealth) technologies are increasingly being employed to deliver interventions to users in their natural environments. With the advent of increasingly sophisticated sensing devices (e.g., GPS) and phone-based EMA, it is becoming possible to deliver interventions at moments when they can most readily influence a person’s behavior. For example, for someone trying to increase physical activity, moments when the person can be active are critical decision points when a well-timed intervention could make a difference. The promise of mHealth hinges on the ability to provide interventions at times when users need the support and are receptive to it. Thus, our goal is to learn the optimal time and intervention for a given user and context. A significant challenge to learning is that there are often only a few opportunities per day to provide treatment. Additionally, when there is limited time to engage users, a slow learning rate can pose problems, potentially raising the risk that users will abandon the intervention. To prevent disengagement, a learning algorithm should learn quickly in spite of noisy measurements. To accelerate learning, information may be pooled across users and time in a dynamic manner, combining a contextual bandit algorithm with a Bayesian random effects model for the reward function. As information accumulates, however, tuning user and time specific hyperparameters becomes computationally intractable. In this talk, we focus on solving this computational bottleneck.