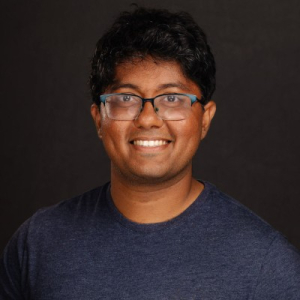
HSPH Biostatistics and DFCI Data Science Colloquium
Monday March 3rd at 4:00pm
HSPH Kresge G2
Adityanarayanan Radhakrishnan
Eric and Wendy Schmidt Center Postdoctoral Fellow, Broad Institute of MIT and Harvard, Harvard School of Engineering and Applied Sciences
Abstract: Understanding how neural networks learn features, or relevant patterns in data, is key to accelerating scientific discovery. In this talk, I will present a unifying mechanism that characterizes feature learning in neural network architectures. Namely, features learned by neural networks are captured by a statistical operator known as the average gradient outer product (AGOP). More generally, the AGOP enables feature learning in machine learning models that have no built-in feature learning mechanism (e.g., kernel methods). I will present two applications of this line of work. First, I will show how AGOP can be used to steer LLMs and vision-language models, guiding them towards specified concepts and shedding light on vulnerabilities in these models. I will then discuss how AGOP can be used to discover cellular programs (sets of genes whose expressions exhibit dependencies across cell subpopulations) from millions of sequenced cells. I will show how AGOP identified programs that reflect the heterogeneity found in various cell types, subtypes, and states in this data. Overall, this line of work advances our fundamental understanding of how neural networks extract features from data, leading to the development of novel, interpretable, and effective methods for use in scientific applications.