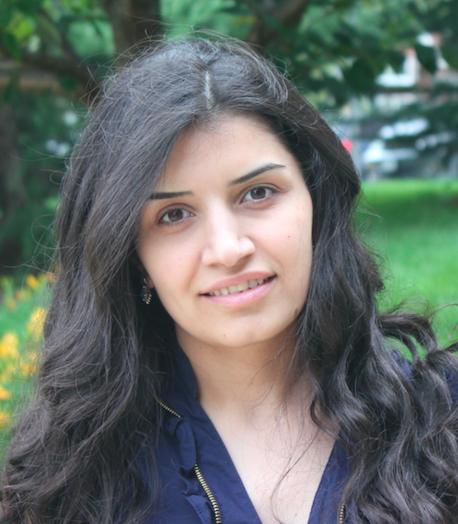
Friday, March 4, 2022
12:00PM Eastern Time
Ani Eloyan, PhD
Assistant Professor
Department of Biostatistics, Brown University
Abstract: Cancer patients routinely undergo radiological evaluations where images of various modalities including computed tomography, positron emission tomography, and magnetic resonance images are collected for diagnosis and for evaluation of disease progression. Tumor characteristics, often referred to as measures of tumor heterogeneity, can be computed using these clinical images and used as predictors of disease progression and patient survival. Several approaches to quantifying tumor heterogeneity have been proposed including simple intensity histogram-based measures, metrics attempting to quantify average distance from a homogeneous surface, and texture analysis-based methods. I will present a statistical framework for principal manifold estimation for obtaining a smooth estimate of tumor surface. I will describe an estimation procedure for tumor heterogeneity using clustering methods considering the neighborhood topology of the tumors. The proposed approach incorporates the spatial structure of the tumor image using neighborhood summary measures. The results of using the proposed methods to estimate cancer survival using machine learning algorithms will be discussed.