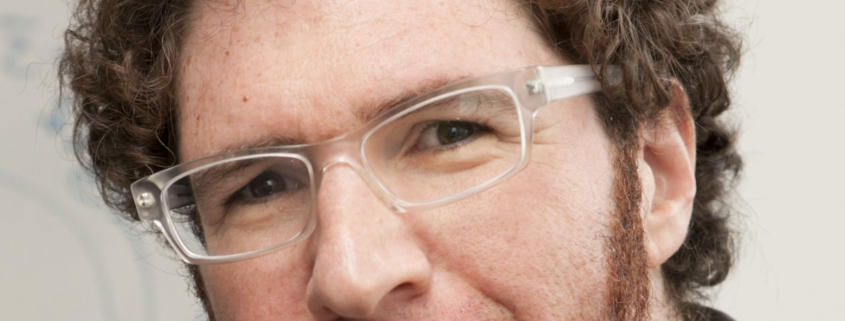
HSPH Biostatistics and DFCI Data Science Colloquium Series
Thursday September 12, 2024
4:00-5:00PM
HSPH FXB Building Room 301
David Blei, PhD
Professor of Statistics and Computer Science
Columbia University
Analyzing nested data with hierarchical models is a staple of Bayesian statistics, but causal modeling remains largely focused on “flat” models. In this talk, we will explore how to think about nested data in causal models, and we will consider the advantages of nested data over aggregate data (such as data means) for causal inference. We show that disaggregating your data—replacing a flat causal model with a hierarchical causal model—can provide new opportunities for identification and estimation.
As examples, we will study how to identify and estimate causal effects under unmeasured confounders, interference, and instruments.
This is joint work with Eli Weinstein.